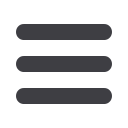
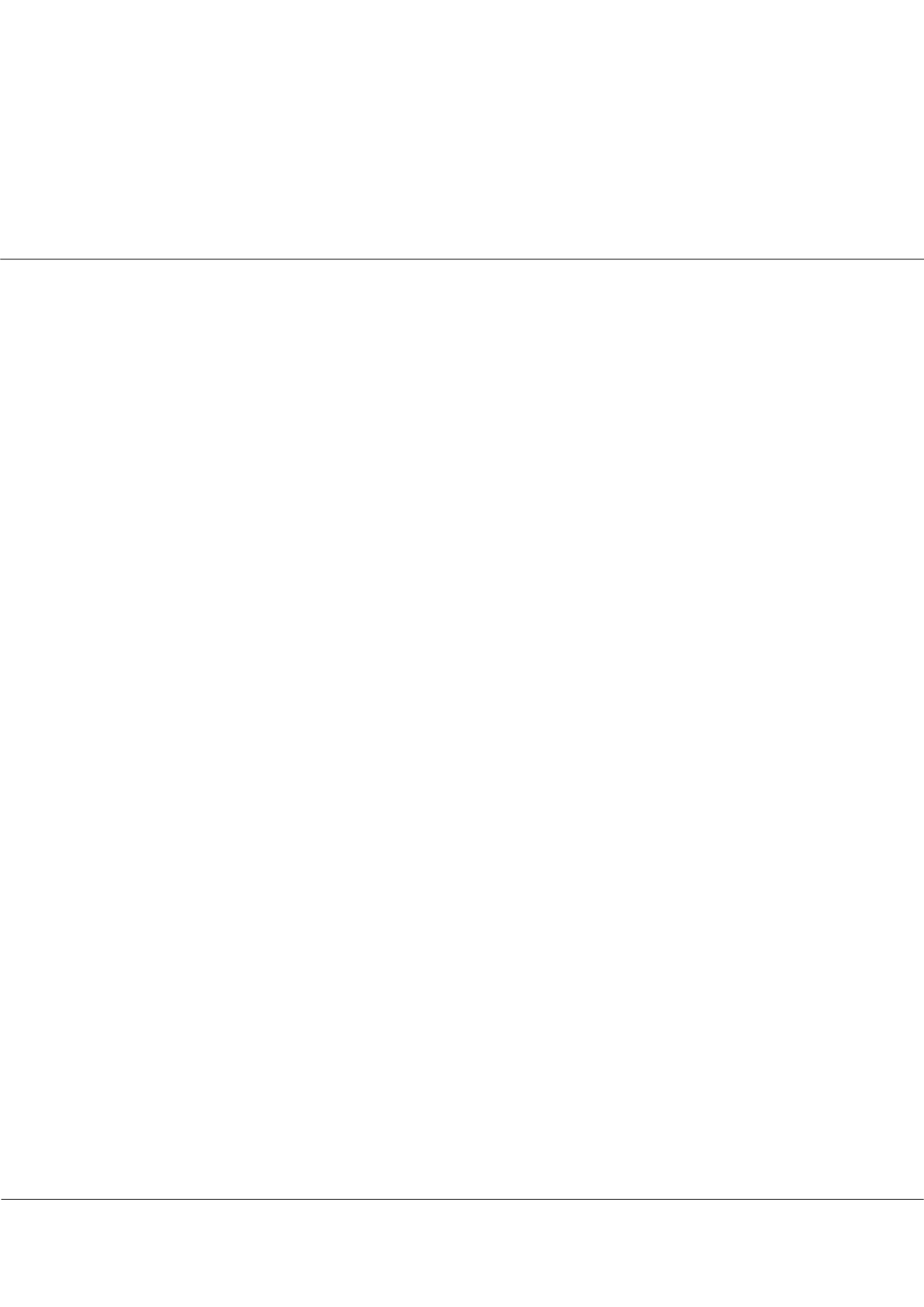
Page 75
conferenceseries
.com
Volume 8
Journal of Biotechnology & Biomaterials
ISSN: 2155-952X
Pharma Biotech 2018
December 10-11, 2018
December 10-11, 2018 | Rome, Italy
23
rd
International Conference on
Pharmaceutical Biotechnology
Modeling and optimization of fermentation conditions for glycolipopeptide production using response
surface methodology and artificial intelligence approaches
Maurice G Ekpenyong
1
, Sylvester P Antai
1
, Atim D Asitok
1
, Rama R Karri
2
and
Bassey O Ekpo
3
1
University of Calabar, Nigeria
2
Universiti Teknologi Brunei, Brunei
3
Nigerian National Petroleum Corporation, Nigeria
Statement of the Problem:
Pseudomonas aeruginosa strain IKW1 produced a biosurfactant when grown in waste frying
sunflower oil-basal medium. The active compound reduced surface tension of fermentation broth to 24.62 dynes/cm at a critical
micelle concentration of 20.80 mg/L. It was identified by high performance liquid chromatography and Fourier transform-
infrared spectrometry as a glycolipopeptide. It demonstrated considerable emulsification and foaming capabilities suggesting
suitability for applications in pharmaceutical and detergent formulations. However, product yield was low, making large-scale
production for recommended applications impracticable. Several researchers have reported yield improvement by strategic
medium optimization approaches. Earlier, we adopted response surface methodology (RSM) for major nutrients optimization
and recorded commendable yield increase. Later on, we employed Placket-Burman design (PBD) and RSM to screen and
optimize trace nutrients and obtained significant yield improvement. However, research reports indicate that artificial neural
network (ANN) is a better optimization approach.
Methodology &Theoretical Orientation:
In this study, we optimized fermentation conditions like temperature, pH, agitation
and duration using RSM, and compared results to those obtained with ANN linked with genetic algorithm (ANN-GA) and
particle swarm optimization (ANN-PSO).
Findings:
Our results showed that the biosurfactant response model, predicted by a quadratic function of RSM, was significant
(P<0.0001; adjusted R
2
=0.9911; RMSE=0.034), setting factor levels at temperature-32°C, pH-7.6, agitation speed-130 rpm and
fermentation time-66 h. Maximum glycolipopeptide concentration was 107.19 g/L with a yield (Yp/x) of 4.24. Comparative
results fromANN-GA (R
2
=0.9997; RMSE=0.055) and ANN-PSO (R
2
=0.9914, RMSE=0.047) showed that model and optimized
factor settings were not significantly (P>0.05) different from those obtained with RSM.
Conclusion&Significance:
This suggests that RSM, whenmeticulously executed, could be as good amodeling and optimization
tool like neural network methods.
maurygg2002@yahoo.comJ Biotechnol Biomater 2018, Volume 8
DOI: 10.4172/2155-952X-C8-110