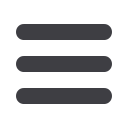
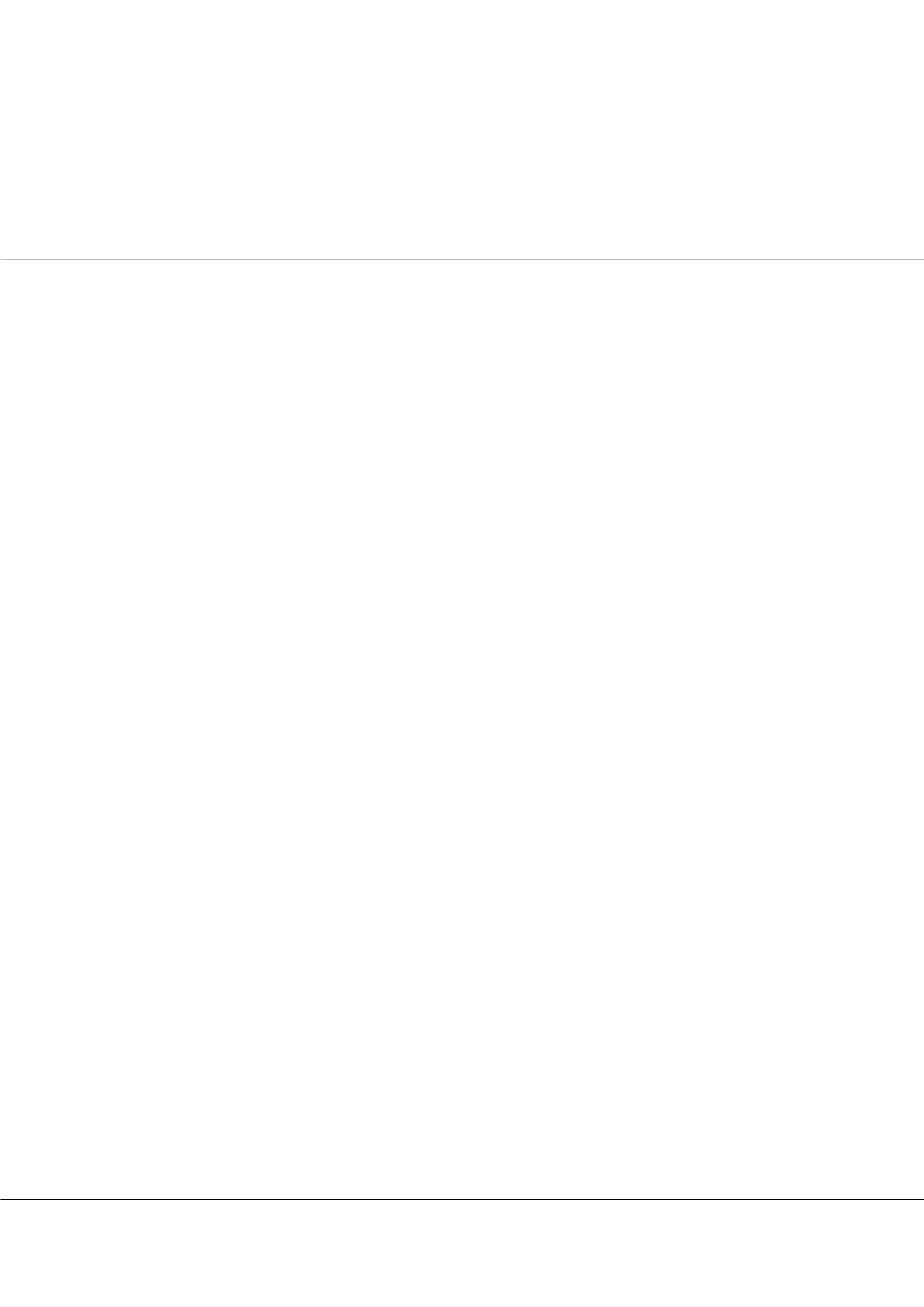
Page 31
Notes:
conferenceseries
.com
Volume 7, Issue 3(Suppl)
J Biotechnol Biomater, an open access journal
ISSN: 2155-952X
Euro Biotechnology 2017
September 25-27, 2017
17
th
EURO BIOTECHNOLOGY CONGRESS
September 25-27, 2017 Berlin, Germany
Assessment of invasive grasses using unmanned aerial vehicles: Amachine learning approach
Felipe Gonzalez
1,2
and
Ed Puig Garcia
1,2
1
Queensland University of Technology, Australia
2
Plant Biosecurity Cooperative Research Centre, Australia
I
nvasive weeds are responsible for irreversible environmental damage, millions of dollars in crop losses and management costs every
year. In order to implement optimal site-specific treatments and reduce control costs, newmethods to accurately monitor and assess
weed and pest damage need to be investigated. In this paper, we explore the combination of unmanned aerial vehicles (UAV), remote
sensing, sensors and machine learning techniques as a promising technology to address this challenge. The deployment of UAVs as
a sensor platform is a rapidly growing field of study for biosecurity and precision agriculture applications. In this experiment, we use
grass as a case study. A data collection campaign was performed at two different locations covered by Buffel grass and other vegetation
(eg. Green Panic, Spinifex scarab). The first site is at cattle farm Chinchilla, QLD, Australia. The Second locations are two sites at Cape
Range National Park, WA. In this study, we describe the UAV platform deployed to collect high-resolution RGB and hyperspectral
imagery as well as the image processing pipeline implemented to create an ortho-image and machine which results in two or five
classifications of the vegetation abundance maps. The aim of the approach is to simplify the image analysis step by minimizing user
input requirements and avoiding the manual data labelling necessary in supervised learning approaches. The methodology presented
in this paper represents a venue for further research towards automated invasive grass assessments and biosecurity surveillance.
Biography
Felipe Gonzalez is an Associate Professor in the Science and Engineering Faculty, Australia and Team Leader for Integrated Intelligent Airborne Sensing Laboratory
at Queensland University of Technology, Australia. He holds a BE (Mech) and a PhD degree from the University of Sydney. His research explores bioinspired
optimization, uncertainty based UAV path planning and UAVs for environmental monitoring. He leads the CRC plant biosecurity project evaluating unmanned aerial
systems for deployment in plant biosecurity and the CRC PB 2135 optimizing surveillance protocols using unmanned aerial systems and developing pest risk
models of buffel grass using unmanned aerial systems and statistical methods. He is a Chartered Professional Engineer and member of professional organizations
including the RAeS, IEEE and AIAA.
felipe.gonzalez@qut.edu.auFelipe Gonzalez et al., J Biotechnol Biomater 2017, 7:3(Suppl)
DOI: 10.4172/2155-952X-C1-076