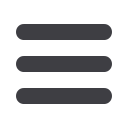
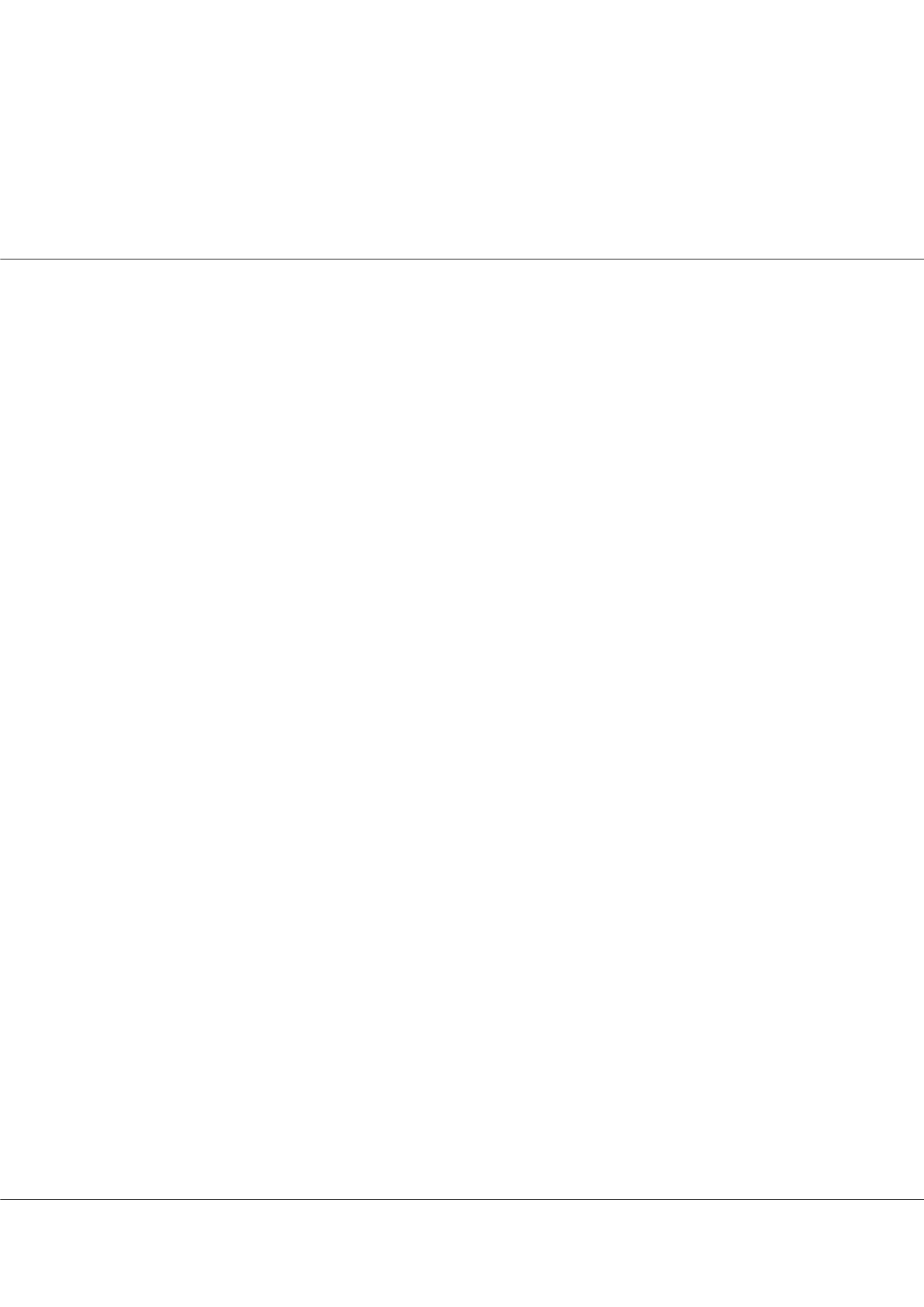
Page 43
Notes:
conferenceseries
.com
Volume 7, Issue 2 (Suppl)
J Adv Chem Eng, an open access journal
ISSN: 2090-4568
Euro Chemical Engineering 2017
November 16-17, 2017
ADVANCES IN CHEMICAL ENGINEERING AND TECHNOLOGY
November 16-17, 2017 | Paris, France
2
nd
International Conference on
Optimization of delay coker unit by using of artificial neural network
Ali Shaeri
NIOEC, Iran
A
Feed forward and multilayer Perceptron Artificial Neural Network (ANN) with back propagation algorithm was applied
for optimization of Delayed Coking Unit (DCU). To this aim, one year of operating conditions were gathered from
Distributed Control System (DCS) and laboratory data. Then all data were evaluated by experienced operators to determine
the most effective operating condition. Conradson Carbon Residue (CCR), asphaltenes content and Feed API were selected
for input of the ANN and the output was set to be maximum products yield. The inputs of neural network data are distributed
for training, validation and testing sets in 60%, 20% and 20% ratios, respectively. A three-layer ANN was adopted to predict
products yield in terms of aforementioned inputs. To find the best fitted ANN structure, several structures (around 1576) were
examined. Here, a total of four combinations of transfer functions were tested against various training functions available in
the MATLAB software. Only the random order incremental training with learning functions (TRAINR) and the Levenberg–
Marquardt (TRAINLM) training functions were found to predict significantly accurate results. It was found out that the best
structure had 4, 6 and 1 neuron for the first, second and third layer, respectively. Furthermore, logsig, logsig and purelin were
found the best transfer function for first, second and third layer. The best model was extracted and the obtained data were
applied to the live DCU with the capacity of 90000 bbl d-1. Transfer functions are generally assigned to a network layer to first
process the input signal, followed by the calculation of suitable weight for the output signal such that the relations of the data
set can be identified. According to many structures which can be used in ANN, an optimization method using correlation
coefficient (R) was recommended to optimize the number of neurons and transfer function types. The results showed that the
selected model could be exactly matched with the DCU.
Biography
Ali Shaeri has a PhD in Process Design Engineer. He is expert in the Refinery’s Process Units Simulation, Process Equipment Design, and Refinery Units
Operation. He is teaching related courses at the university as well. On the other hand, He is expert in the optimization, new method of mini-refinery design and
related pilot plant design additionally. He is working in the National Iranian Oil Engineering & Construction Co. from 11 years. He obtained B.S. degree in Process
Design Engineering in 2002,
M.Sc. degree in Process Design Engineering in 2006 and PhD degree in Process Design Engineering in 2016.Since then he joined
Process Specialty Committee and permanent member of Iranian Petroleum Standard from 2007 to present. He is the first designer of Mini Refinery in Iran and head
of Process Department for Petroleum Desalting Project. He has to his credit more than 10 Technical Reports and proposals related to Process Design, as well as,
30 publications including papers in referred journals, books and conferences papers.
a.shaeri@iau-tnb.ac.irAli Shaeri, J Adv Chem Eng 2017, 7:2(Suppl)
DOI: 10.4172/2090-4568-C1-002