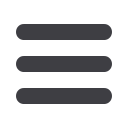
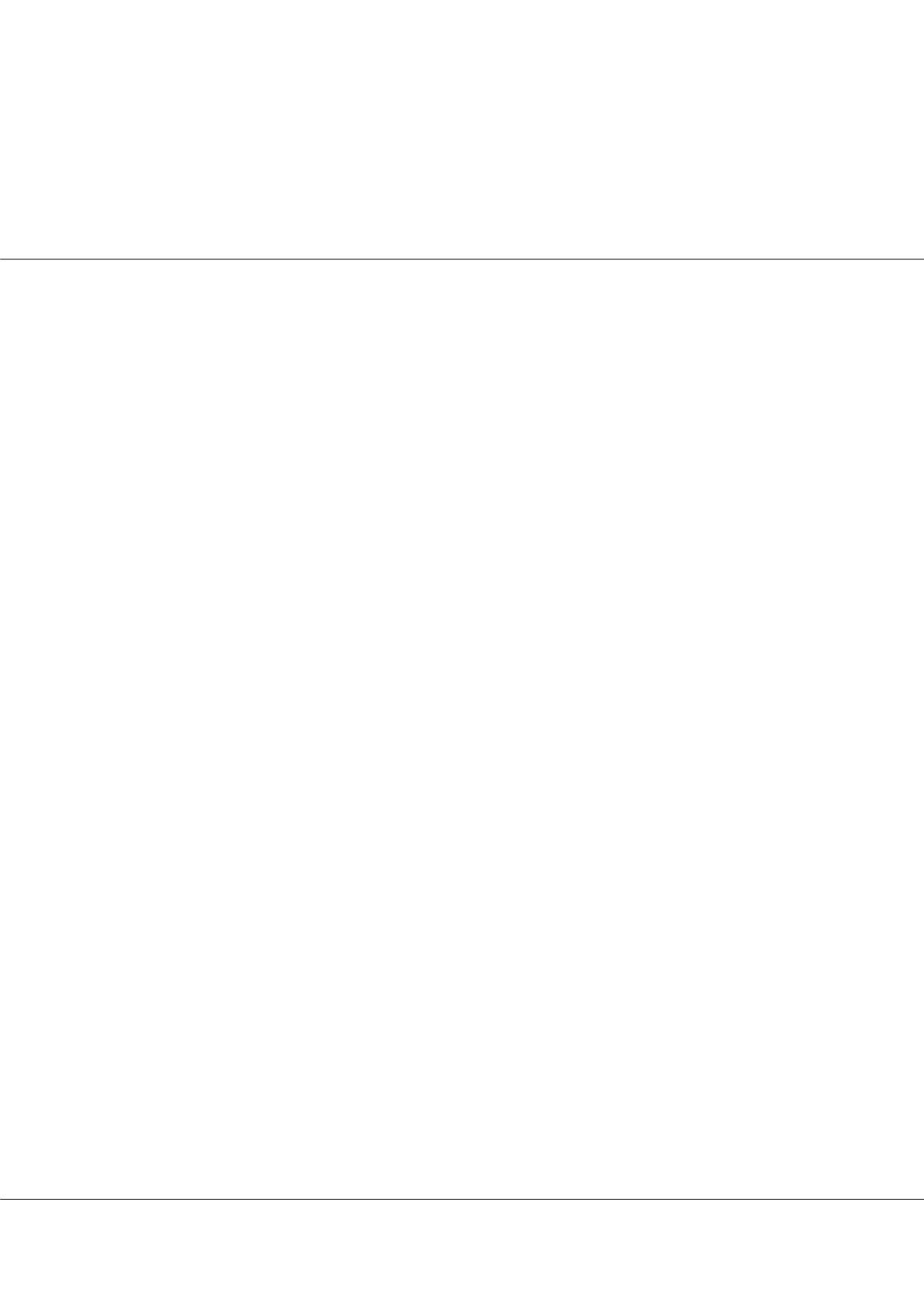
Page 25
Journal of Ecosystem & Ecography | ISSN: 2157-7625 | Volume: 8
June 28-29, 2018 | Alexandria, Egypt
International Pre Conference Workshop on
Microbial Ecology & Eco Systems
Detection of mango leaf spots andmealybugs diseases using deep learning “ConvNet” and LinearSVC
Mohamed S Aboalarbe
and
Ammar Adl
Beni-Suef University, Egypt
L
eaf Spots and Mealybugs lesion infected a lot of plants of the local city of Beni-Suef. In this proposed paper, pre-trained
ConvNet was combined with LinearSVC and some image processing techniques in the process of Detection of Leaf Spots
and Mealybugs lesion at mango to enables the farmers to save their crops and improve the quality and the quantity. This
research is done to achieve of detection of these two diseases at mango and predict a treatment according to each disease
with accuracy of 97% in the ordinary lighting conditions using a high-quality photo or mobiles camera. This paper covers
the detection process using machine learning and deep learning algorithms; LinearSVC, ConvNet and image processing
techniques; noise removal filters, resizing the images. The proposed solution achieved the detection of these two diseases and
methods of treatment with accuracy of 97%. The proposed system is built over state of the art machine learning, deep learning,
transfer learning and image processing used for learning process of the computer vision. It is developed by combining these
factors: (A) Pre-processing, (B) Features Extraction, (C) Classification, (D) Testing and Evaluating. The effect of using transfer
learning on the processing time and accuracy: When we build a ConvNet from scratch, we need a very large amount of data
and a lot of time-consuming to training the network. So we apply the concept of transfer learning that pre-train the ConvNet
on a very large dataset e.g. ImagNet which contains about 1.2 million images with 1000 categories then we will use the ConvNet
as a fixed features extractor and this led to decreasing the processing time. Features that extracted from pre-trained ConvNet
are very relevant and powerful for awesome classification results and this led to increasing the model`s accuracy.
Keywords:
Deep Learning, Image processing, Leaf spots Mealybugs, Mango Diseases.
Biography
Mohamed Aboalarbe is a researcher and problem solver who uses computer science-based approach to new challenges. He is passionate about development
and applying software engineering concepts and principles, and committed to maintaining cutting edge technical skills and up-to-date industry knowledge. Over
the last three years, he gained an extensive experience in software engineering, and training programs relevant to the field. He is particularly interested in Android
development and applications using different technologies such as Java, XML, Firebase, Google Cloud, third party libraries, responsive screens, Material Design,
Gradle Scripts, Nodes js, Web Services, REST, etc.
mohamedaboalarbe@fcis.bsu.edu.egMohamed S Aboalarbe et al., J Ecosys Ecograph 2018, Volume: 8
DOI: 10.4172/2157-7625-C2-035