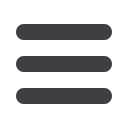
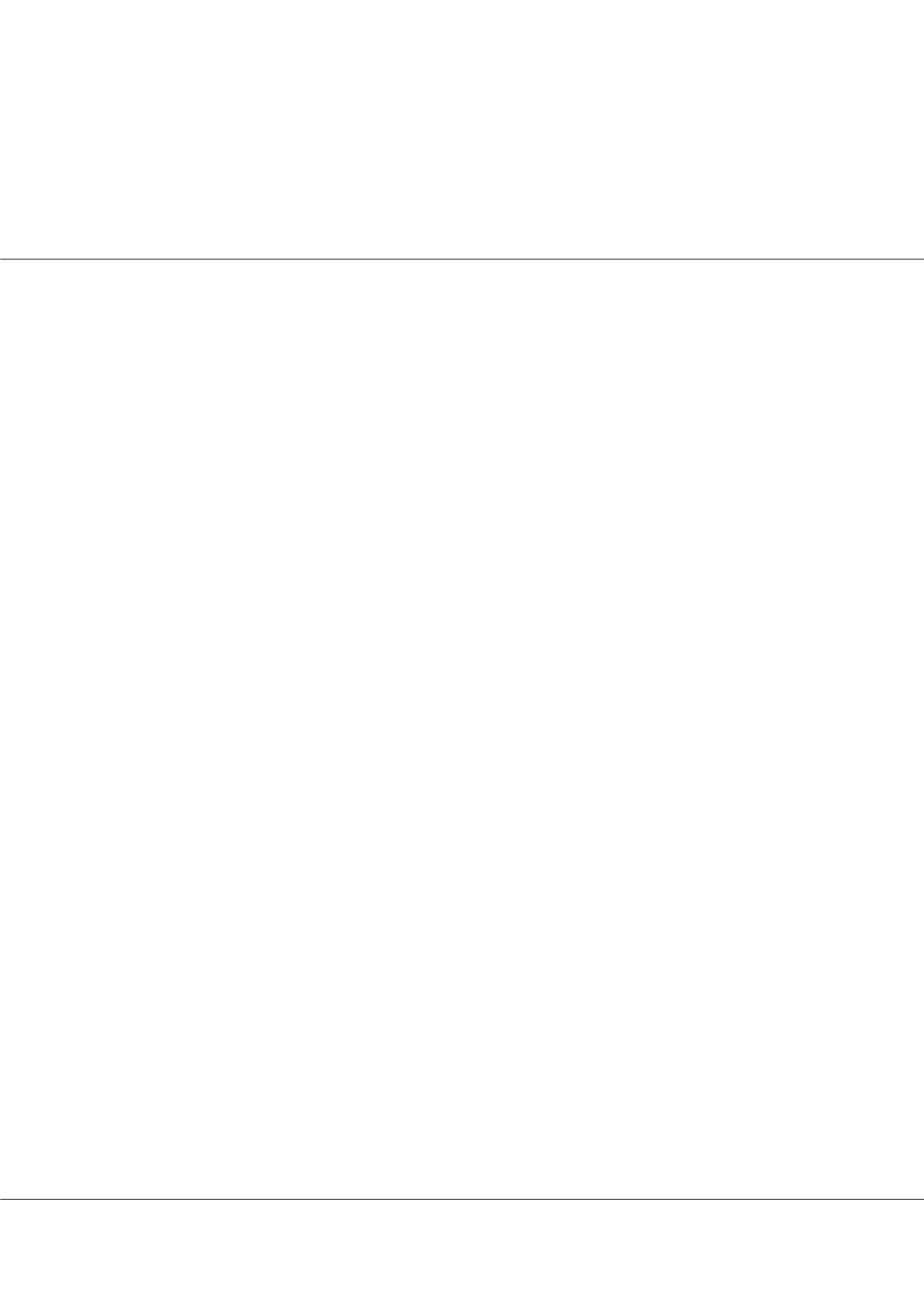
Page 56
Notes:
Journal of Clinical & Experimental Pathology | ISSN: 2161-0681 | Volume 8
December 03-04, 2018 | Chicago, USA
American Pathology and Oncology Research
&
15
th
International Congress on
Microbial Genetics and Molecular Microbiology
International Conference on
Automated segmentation of nucleus, cytoplasm, and background of pap-smear images using a trainable pixel
level classifier
Wasswa William
1
, Andrew Ware
2
, Annabella Habinka Basaza-Ejiri
3
and
Johnes Obungoloch
1
1
Mbarara University of Science and Technology, Uganda
2
University of South Wales, UK
3
St. Augustine International University, Uganda
Background:
Cervical cancer ranks as the fourth most prevalent cancer affecting women worldwide and its early detection provides
the opportunity to help save a life. Automated diagnosis and classification of cervical cancer from pap-smear images has become
a necessity as it enables accurate, reliable and timely analysis of the condition’s progress. Segmentation is a fundamental aspect of
enabling successful automated pap-smear image analysis. In this paper, a potent algorithm for segmentation of the pap-smear image
into the nucleus, cytoplasm, and background using pixel level information is proposed.
Methods:
First, a number of pixels from the nuclei, cytoplasm, and background are extracted from five hundred images. Second, the
selected pixels are trained using noise reduction, edge detection, and texture filters to produce a pixel level classifier. Third, the pixel
level classifier is validated using test set and 5- fold cross validation using Fast Random Forest, Naïve Bayes, and J48 classification
techniques.
Results:
An extensive evaluation of the algorithm and comparison with the benchmark ground truth measurements shows promising
results. Comparison of the segmented images’ nucleus and cytoplasm parameters (nucleus area, longest diameter, roundness,
perimeter and cytoplasm area, longest diameter, roundness, perimeter) with the ground truth segmented image feature parameters
(nucleus area, longest diameter, roundness, perimeter and cytoplasm area, longest diameter, roundness, perimeter) yielded average
errors of 0.94, 0.93, 0.02, 0.63, 0.96, 0.37, 0.13 and 0.96mm respectively. Validation of the proposed pixel level classifier with 5-fold
cross-validation yielded a classification accuracy of 98.48%, 94.25% and 98.45% using Fast Random Forest, Naïve Bayes, and J48
classification methods respectively. Finally, validation with a test dataset yielded a classification accuracy of 98.48% and 98.98% using
Fast Random Forest and J48 Classification methods respectively.
Conclusion:
This paper articulates a potent approach to the segmentation of cervical cells into the nucleus, cytoplasm, and
background using pixel level information. The experimental results show that the approach gives good classification and achieves
a pixel classification average accuracy of 98%. The method serves as a basis for first level segmentation of pap-smear images for
diagnosis and classification of cervical cancer from pap-smear images using nucleus and cytoplasm pixel level information.
wwasswa@must.ac.ugJ Clin Exp Pathol 2018, Volume 8
DOI: 10.4172/2161-0681-C5-058