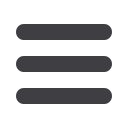
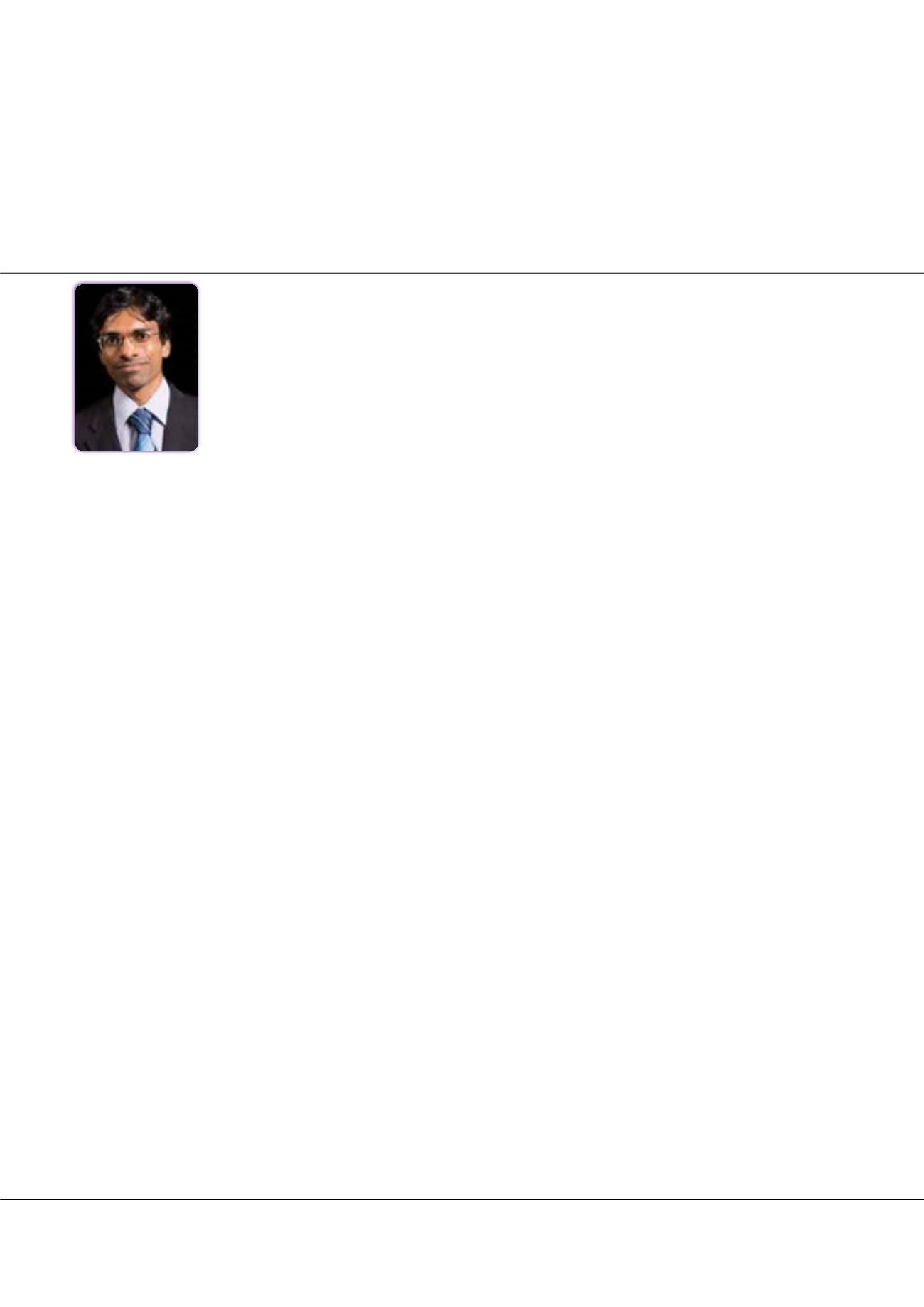
Volume 4, Issue 4(Suppl)
J Infect Dis Ther 2016
ISSN: 2332-0877, JIDT an open access journal
Infectious Diseases 2016
August 24-26, 2016
Page 74
Notes:
conference
series
.com
August 24-26, 2016 Philadelphia, USA
&
Infectious Diseases
Joint Event on
2
nd
World Congress on
Pediatric Care & Pediatric Infectious Diseases
International Conference on
Lalit Garg, J Infect Dis Ther 2016, 4:4(Suppl)
http://dx.doi.org/10.4172/2332-0877.C1.007Infectious disease progression modeling
M
odels which can predict disease progression are useful for aiding clinicians in prescribing the correct treatment at the
optimal time to produce the best outcome for the patient. Positive correlations between changes in a patient’s infection
state with respect to other factors of the patient’s profile such as age, gender and treatment. We utilize artificial neural networks
and phase type survival trees with differing combinations of input covariates to find which ones provide the best predictor of
the future state. To demonstrate the model, we used a dataset of 1,838 patients infected with the human immunodeficiency
virus (HIV) which were enrolled in the Italian public structures between January 1996 and January 2008. The proposed
disease progression models effectively cluster, identify and quantify the effects of these covariates and their interaction in the
prediction of HIV disease progression. Our results show that antiretroviral treatment (ART) is the best prognosticator of a
patient’s future state followed by the CD4+ T-lymphocyte measurement. Other covariates such as gender and age have little
impact on the overall accuracy in prediction. Results improved dramatically when predicting if the patients’ next state was
AIDS (Acquired immunodeficiency syndrome). These results should aid in the management of HIV and its treatment while
the methods developed through this research can also be useful for modeling disease progression in patients who have other
chronic conditions or diseases such as tuberculosis (TB), the severe acute respiratory syndrome (SARS), cardiovascular disease
(CVD), cancer and diabetes.
Biography
Lalit Garg has received his PhD degree from the University of Ulster, UK in 2010. He has received his first degree in Electronics & Communication Engineering
from the Barkatullah University, India in 1999 and Postgraduate degree in Information Technology from ABV-IIITM, Gwalior, India in 2001. He is currently a Senior
Lecturer in Computer Information Systems at the University of Malta, Malta. He was a Researcher at the Nanyang Technological University, Singapore and at the
University of Ulster, UK. His research interests include missing data handling, machine learning, data mining and their applications especially in the healthcare
domain. He has published more than 60 technical papers in refereed journals and conferences.
lalit.garg@um.edu.mtLalit Garg
University of Malta, Malta