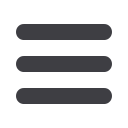
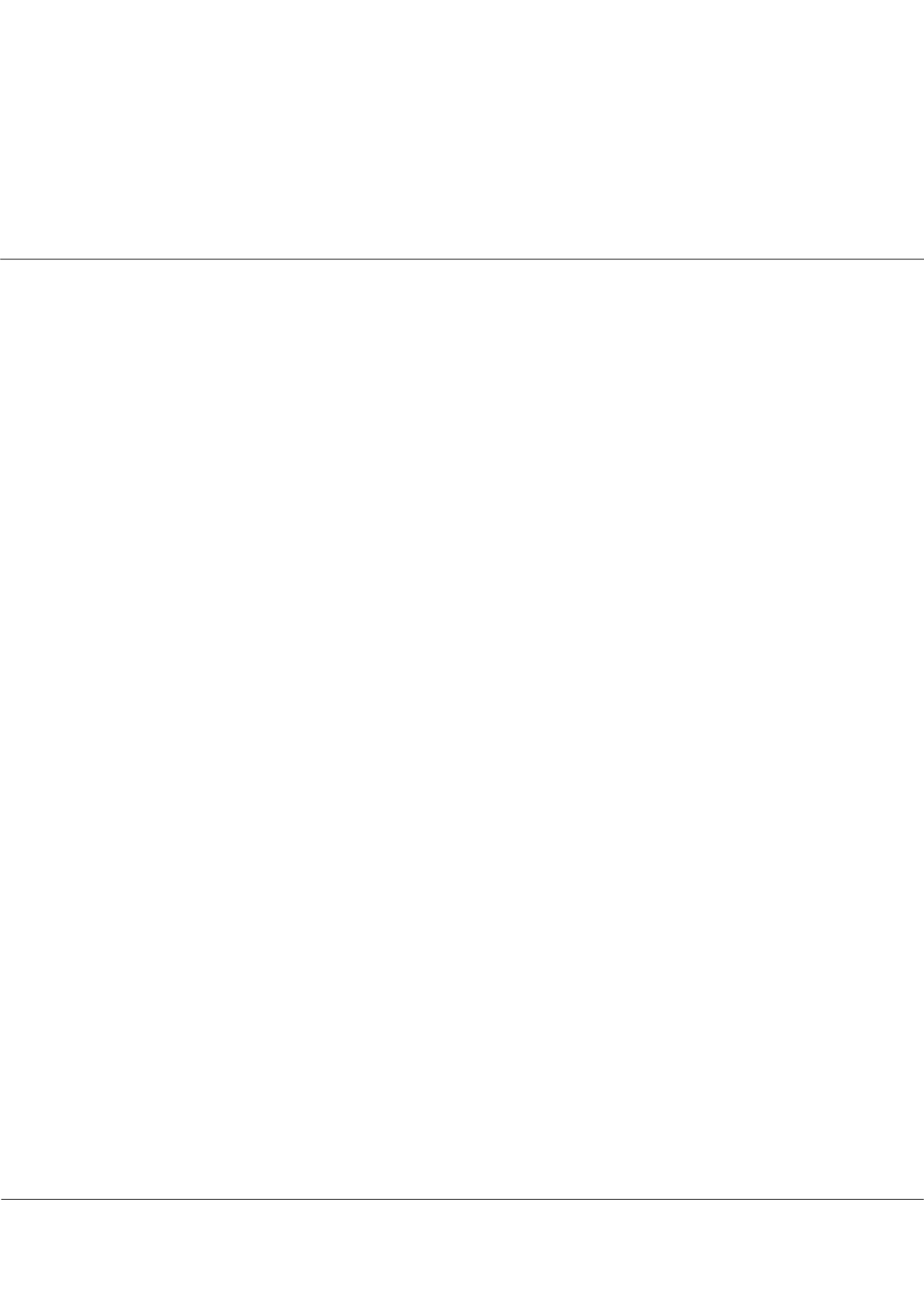
Page 73
Notes:
conferenceseries
.com
Volume 8
Epidemiology: Open Access
ISSN: 2161-1165
Epidemiology 2018
September 17-19, 2018
September 17-19, 2018 | Rome, Italy
8
th
International Conference on
Epidemiology & Public Health
Dynamical augment in mining healthcare datasets
John Heironimus
1
and
Emma Heironimus
2
1
Fleming Scientific, USA
2
University of Louisville, Kentucky
S
tatistical processes commonly applied to healthcare datasets can overlook important dynamical relationships. However,
understanding dynamicals often involves complex and expensive modelling. We demonstrate a simple approach to
“dynamical data mining” using Fourier transform and the Kuramoto model. We compare results from this approach to cross
correlations using CDC’s ICD-113 data (1999-2016). We find that the ICD-113 is a synchronous anti-phase nodal system
much like the spontaneous synchrony of pendulum clocks observed by Christiaan Huygens in 1665 (see image). It is a state
of order in which ICDs are related phasallyabout common frequencies. We find that about 20% of these phasalrelationships
are novel to the statistical approach and corroborated by research findings. Many of these novel insights appear to be complex
time series relationships suggesting stronger linkages between initial conditions/care and final causes of death than statistics
would typically reveal. ICD-113’s state of order remains stable even as the mix of ICDs changes. This suggests that declining
US healthcare productivityis perhaps more attributable to complex dynamical relationships within the system than to rising
obesity or opioid abuse rates as often hypothesized. Given the system’s stablestate of order, we can simulate the impact of
changing incidence of any one condition on all others without consideration of causality. As a synchronous system can reflect
the influence of external factors, we also consider the possible influence of solar cycles. Using the SILSO dataset, we find
consistency with ICD-113 (see image). Each ICD’s phasalrelationship to individual solar cycles allows us to infer its most
likely time series relationship and possibly forecast future incidence on the same basis. We offer several detailed examples of
all points in our paper and conclude that simple “dynamical mining” offers an important augment to statistical processing of
healthcare datasets.
Biography
John Heironimus founded Fleming Scientific to develop novel dynamical approaches to understanding human populations where said offers augmentation to
statistical and cognitive processes typically employed. FS has successfully characterized a wide variety of social and commercial populations based on the notion
that stability accrues from states of order, in some dimensionality, that are the steady state solutions of dynamical processes. He holds degrees from Harvard and
the University of Pennsylvania. Emma Heironimus assisted in the development of the above paper and is a dual-enrollment student at UoL where she participates
in trauma research.
jh@flemingscientific.comJohn Heironimus et al., Epidemiology (Sunnyvale) 2018, Volume 8
DOI: 10.4172/2161-1165-C1-020