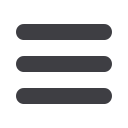
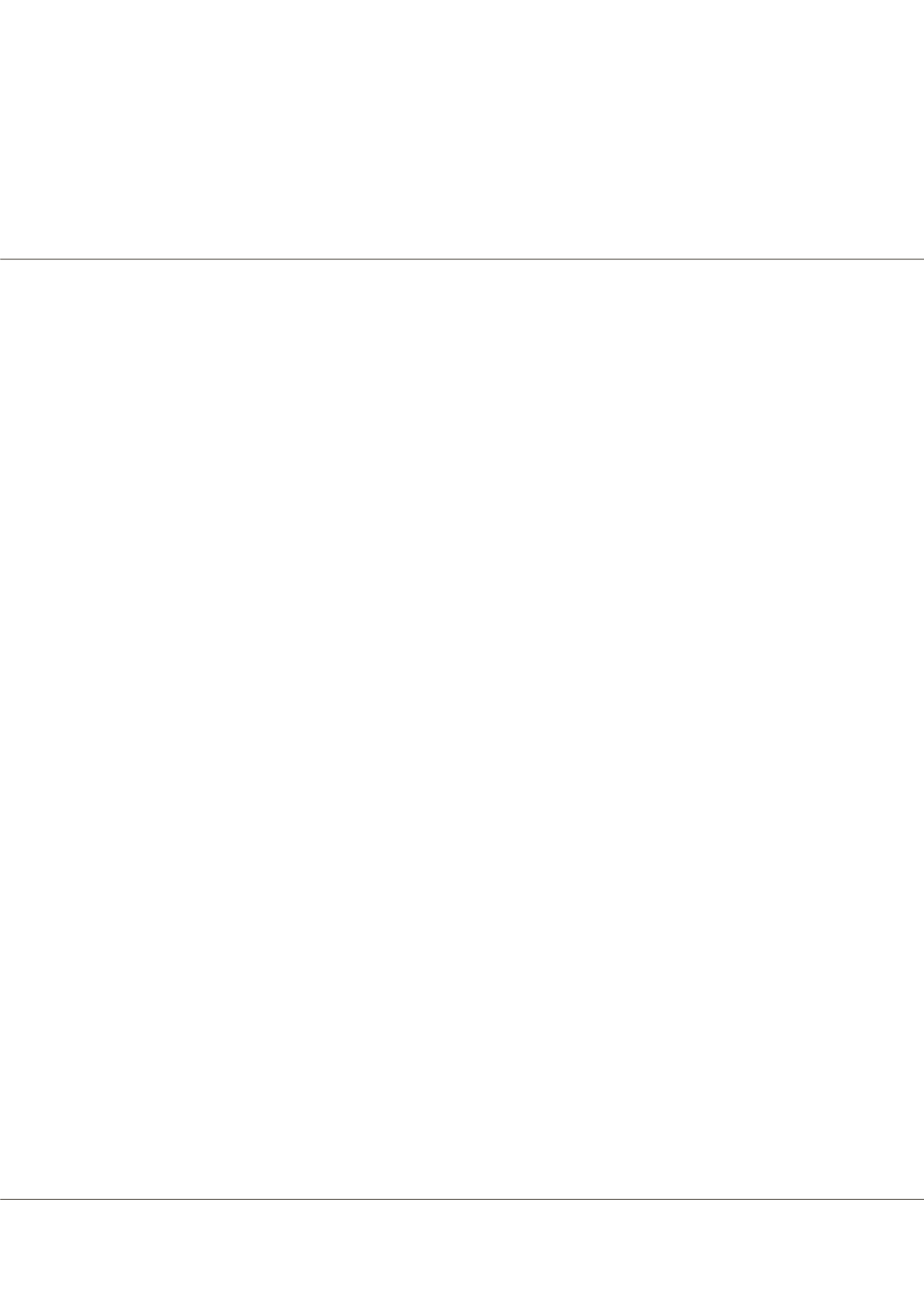
Page 44
conferenceseries
.com
Volume 7, Issue 4 (Suppl)
J Nephrol Ther 2017
ISSN: 2161-0959 JNT, an open access journal
Nephrology & Urology 2017
July 06-07, 2017
JULY 06-07, 2017 KUALA LUMPUR, MALAYSIA
12
TH
ANNUAL CONFERENCE ON
Nephrology & Urology
What changes the quality of life in a hemodialysis patient - A machine learning approach
Shoab Saadat
Shifa International Hospital, Pakistan
Statement of the Problem:
Lifestyle of hemodialysis patients has a significant impact on their quality of life (QOL). Physical,
psychological, social, environmental, and financial factors play an important role in determining the QOL. Several studies
identify the most significant correlates with a better QOL in these patients. Because, there has been no study specifically aiming
at predicting a change in QOL using modern machine learning techniques, therefore, the purpose of this study is to produce a
classification model for the most important positive and negative predictors for the QOL in hemodialysis patients.
Methodology & Theoretical Orientation:
This is a prospective cohort study of patients on at least 3 months of hemodialysis.
By the first interim analysis, a total of 78 patients were administered a proforma containing questions about demographics and
the validated Urdu version of WHO BREF questionnaire for the QOL assessment by a MBBS qualified doctor on day 0 and 30.
Statistical analysis was performed using SPSS version 24, while machine learning algorithms including the classification tree were
generated using Orange.
Findings:
A total of 78 patients were enrolled and analyzed for the first interim analysis (42 males, 36 females). The domain means
of WHO BREF questionnaire for QOL were: Physical=12.9 (SD=3.7), Psychological=15.0 (SD=3.4), Social=15.2 (SD=2.75),
Environmental=16 (SD=2.9) respectively. Linear regression model (p<0.000, R2=0.418), showed monthly income (p<0.000) and
serum albumin (p<0.000) to be positively and significantly associated with better QOL. Among machine learning algorithms
(classification tree and Naïve Bayes models), classification tree was the most accurate (AUC=83.3%).
Conclusion & Significance:
Machine learning algorithms can be used to classify patients into those with higher probabilities
of having a given change in QOL in future. This can in turn be used to risk stratify patients and for better utilization of health
resources.
dr.shoaibsaadat@gmail.comRenal amyloidosis: An update and focus on newly described entities
Md. Shahrier Amin
Arkana Laboratories, USA
A
myloidosis is a systemic protein folding disease where insoluble 7-12 nm fibrils having a β-pleated sheet architecture are
deposited in the extracellular space in different tissues. Depending on the amyloid precursor protein, there are different
types of systemic and organ specific amyloidosis. Differentiating between the different types is crucial, because subsequent
management depends on the type and extent of the amyloidosis. When the kidneys are involved, patients often present with
proteinuria. Amyloidosis is seen in up to 5% of adult patients with nephrotic syndrome. Proper interpretation of findings on
a kidney biopsy is crucial. Pathologic diagnosis requires special stains, immunofluorescence and electron microscopy. Mass
spectrometry is sometimes necessary for definite characterization of some rare types of amyloidosis and is an eligibility criterion
for targeted therapy in some clinical trials. Immunoglobulin light chains (AL) and serum amyloid A protein (AA) form the
basis of most common forms of amyloidosis, accounting for up to 90% of cases. While AL amyloidosis is often associated with
lymphoproliferative disorders, AA amyloidosis is commonly seen with chronic inflammatory disorders including infections.
Several other amyloidogenic proteins have recently been described and associated with particular histopathologic features. These
include leukocyte chemotactic factor 2 (ALECT2), apolipoprotein A-IV and gelsolin. LECT2 amyloidosis is particularly seen in
patients of specific ethnicities. Apolipoprotein A-IV amyloidosis shows a peculiar predilection to deposition in the medulla. This
lecture will help to educate the audience about common forms of amyloidosis and gain further insight about newly described
entities.
mdshahrier@gmail.comJ Nephrol Ther 2017, 7:4 (Suppl)
DOI: 10.4172/2161-0959-C1-043