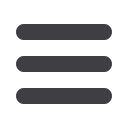
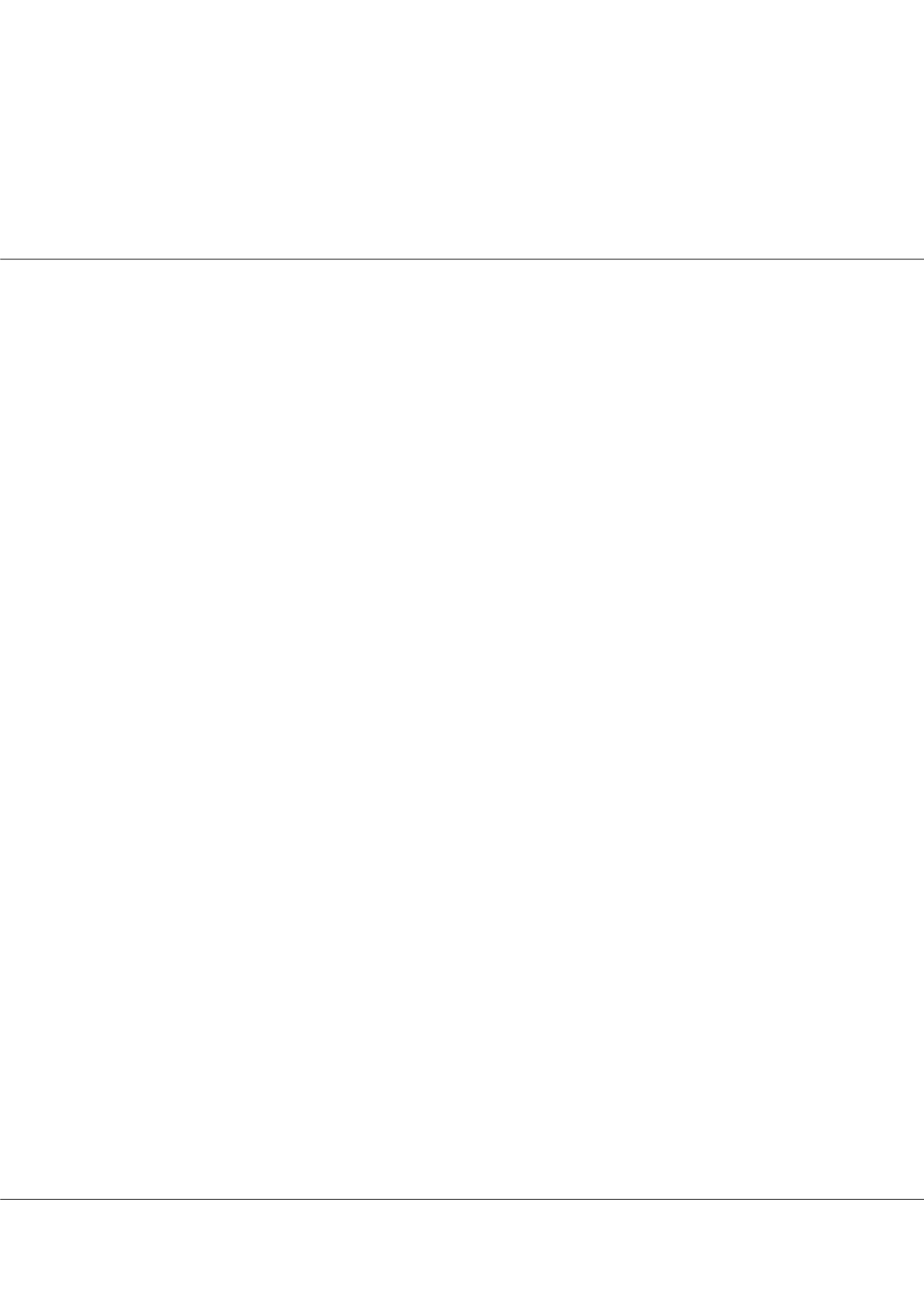
Page 60
Notes:
Industrial Chemistry | ISSN: 2469-9764 | Volume 4
17
th
International Conference on
May 21-22, 2018 | New York, USA
Industrial Chemistry and Water Treatment
A long-term aerosol prediction model based on a bi-directional long-short term memory neural
network
Inyoung Park, Jiwon Lee, Hyun Soo Kim, Chul Han Song
and
Hong Kook Kim
Gwangju Institute of Science and Technology, Korea
W
ith increasing of interests in aerosol for national environmental crisis, prevention of aerosol becomes a major issue
for human-being health. As the ecosystem vulnerability becomes clear, the need for long-term aerosol prediction has
been attracted. Since it is known that the amount of aerosol is highly related to pollutants such as CO, SO
2
, NO, and so
on, the accuracy of long-term aerosol prediction can be increased by exploiting the relation between pollutants and aerosol
levels. Therefore, this paper proposes a long-term aerosol prediction model based on a bi-directional long-short term memory
(B-LSTM) network, which is a well-known deep neural network technique applied to time-series data. The proposed model
is composed of two layers of the B-LSTM network. The lower layer is designed to predict a pollutant rate for up to 3-hour by
using the correlation between pollutants and aerosol. The upper layer of the B-LSTM network is for predicting a PM10 rate up
to 24-hours by using the lower layer outputs. Here, the B-LSTM network is trained using actual pollutant data collected on an
hourly basis for 30 years (from 1987 to 2016) of 15 different industrial locations of South Korea. The prediction accuracy of the
lower layer of the B-LSTM network achieved 77.4% for 3-hour prediction of pollutants such as CO, SO
2
and NO. In addition,
the prediction accuracy of PM10 from the upper layer of the B-LSTM was evaluated by measuring the root-mean-squared
error (RMSE) between actual and predicted. As a result, the RMSE averaged over 15 locations was measured as about 13.77%
for 24-hour PM10 prediction.
Biography
Inyoung Park is currently pursuing her PhD in the School of Electrical Engineering and Computer Science, Gwangju Institute of Science and Technology, South
Korea. She has received her BS degree in Computer Application from the Bangalore University in 2015. Her current research focuses on speech signal processing
and climate change modeling based on deep neural networks.
pinyoung@gist.ac.krInyoung Park et al., Ind Chem 2018, Volume 4
DOI: 10.4172/2469-9764-C1-009