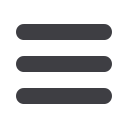
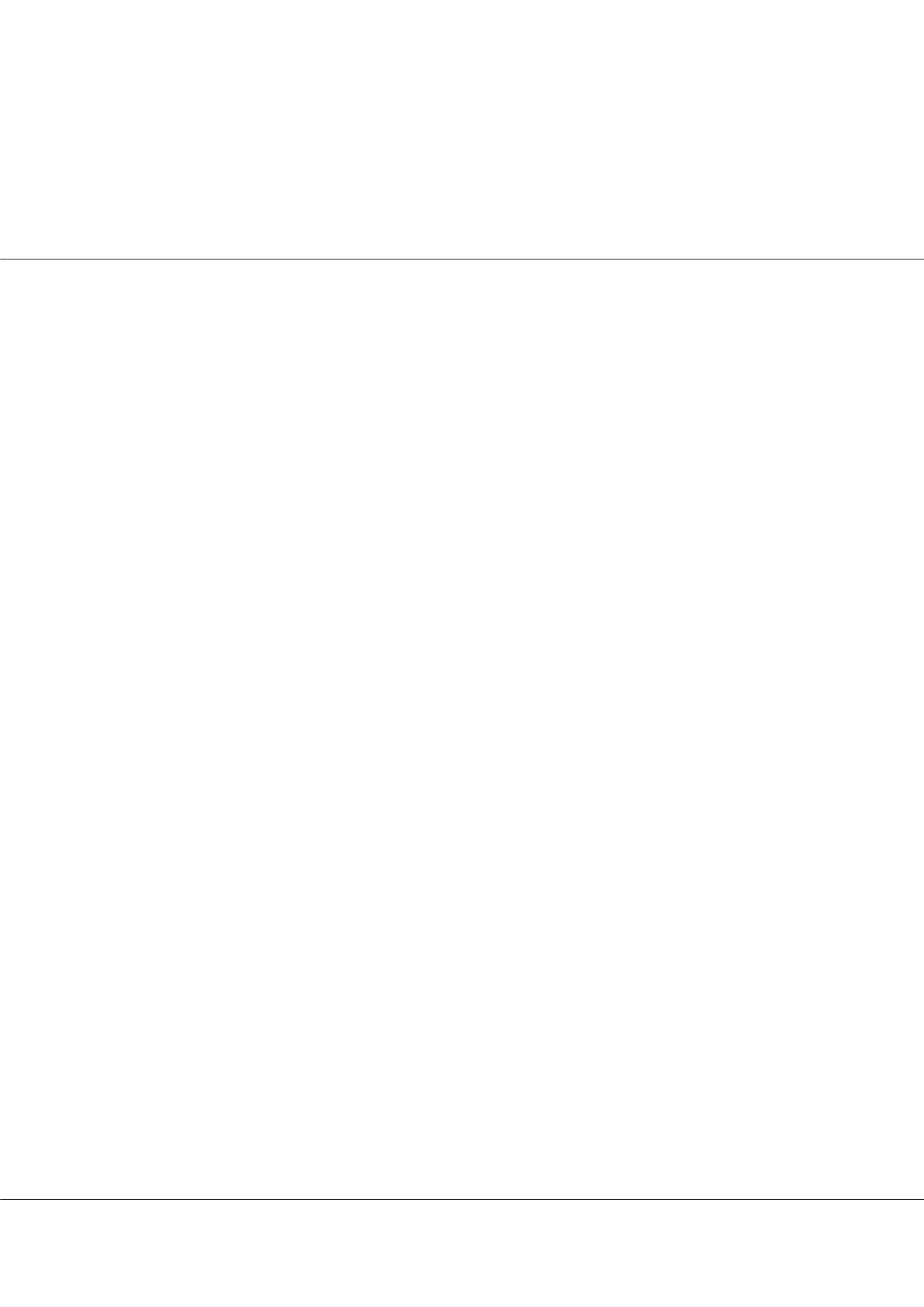
Page 28
Notes:
conferenceseries
.com
Volume 7, Issue 6 (Suppl)
J Clin Exp Pathol, an open access journal
ISSN:2161-0681
Digital Pathology 2017
November 15-16, 2017
November 15-16, 2017 San Antonio, USA
2
nd
International Conference on
Digital Pathology & Image Analysis
Study on the performance of an artificial intelligence system for image based analysis of peripheral
blood smears
Renu Ethirajan, Dheeraj Mundhra, Jaiprasad Rampure, Shreepad Potadar, Sukrit Mukherjee
and
Bharath Cheluvaraju
SigTuple Technologies Pvt Ltd., India
I
n this study, we evaluate SHONIT, a cloud based Artificial Intelligence (AI) system for automated analysis of images captured
from peripheral blood smears. SHONIT’s performance in classification of WBCs was evaluated by comparing SHONIT’s
results with hematology analyzers and manual microscopy for manually stained smears. The study was carried out over 100
samples. The cases included both normal and abnormal samples, wherein the abnormal cases were from patients with one or
more quantitative or qualitative flagging. All the smears were created using Hemaprep auto-smearer and stained using May
Grunwald Geimsa stain. They were scanned and analyzed by SHONIT for WBC differentials under 40X magnification. WBC
morphological classification by SHONIT was verified by an experienced hematopathologist. Quantitative parameters were
analysed by computing the mean absolute error of the WBC DC values between SHONIT and Sysmex XN3000 and between
SHONIT and manual microscopy. The mean absolute error between WBC differential values of manual microscopy and
SHONIT were 7.67%, 5.93%, 4.58%, 2.69%, 0.44% for neutrophil, lymphocyte, monocyte, eosinophil and basophil respectively.
The mean absolute error between WBC differential values values of Sysmex XN3000 and SHONIT were 8.73%, 5.55%, 3.63%,
2.12%, 0.45% for neutrophil, lymphocyte, monocyte, eosinophil and basophil respectively. SHONIT has proven to be effective
in locating and examiningWBCs. It saves time, accelerates the turnaround-time and and increases productivity of pathologists.
It has helped to overcome the time-consuming effort associated with traditional microscopy.
Biography
Renu Ethirajan has completed her MBBS and DNB Pathology from Father Muller Medical College, Mangalore, India. She is currently working as Director
Pathology for SigTuple, an organization that provides healthcare solutions driven by artificial intelligence and image processing. She has worked as a Consultant
Hemato-Oncopathologist and has reported flowcytometry for more than 8 years at HCG Cancer Hospital, Bangalore. She is also trained in molecular diagnosis
like fluoroscent in-situ hybradization and immuno-hematology. She has presented multiple papers in reputed CMEs and conferences. She has participated at the
National Indian Conclave as a panelist on AI.
renu@sigtuple.comRenu Ethirajan et al., J Clin Exp Pathol 2017, 7:6 (Suppl)
DOI: 10.4172/2161-0681-C1-043