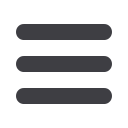
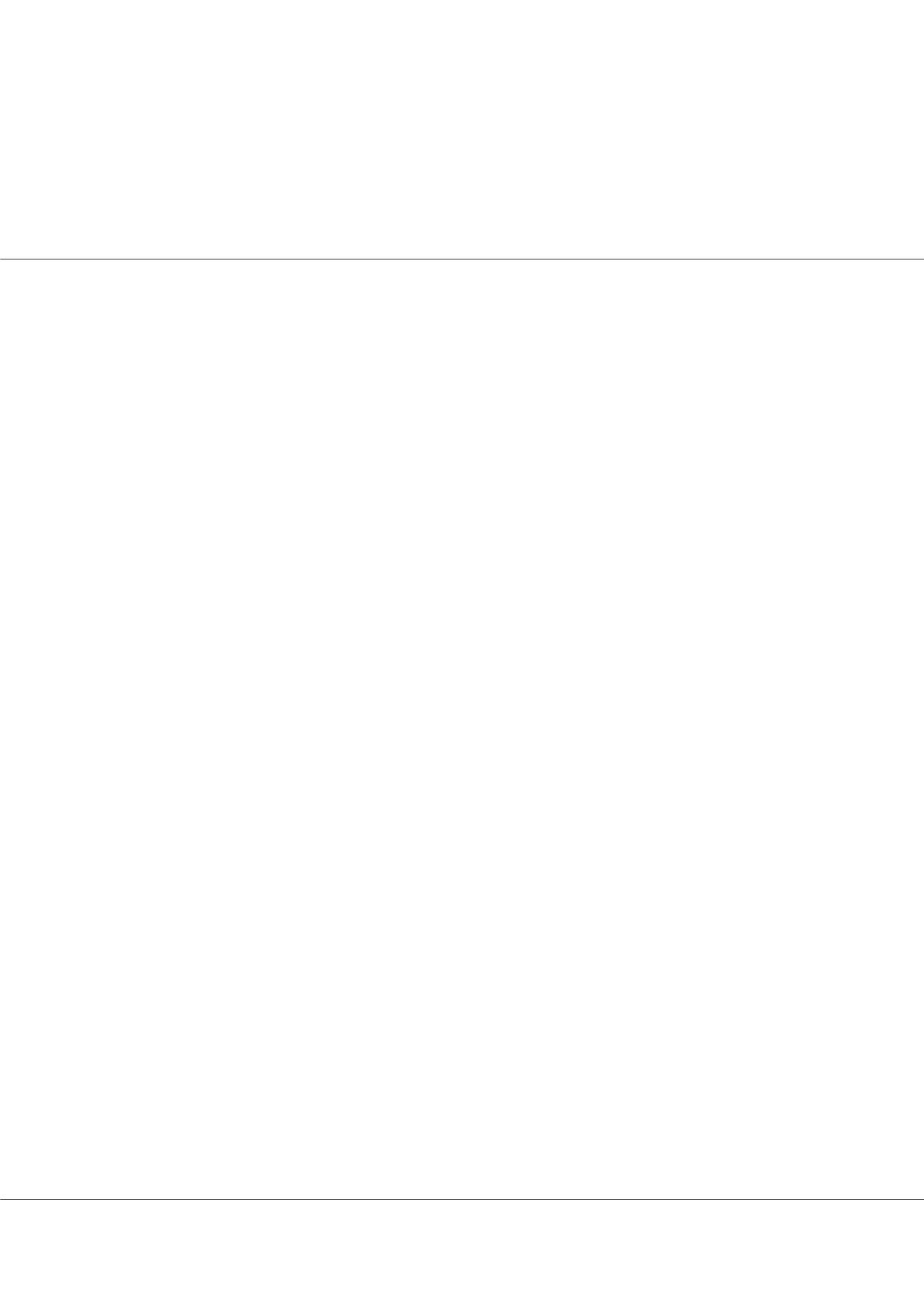
Page 26
conferenceseries
.com
Volume 5, Issue 3 (Suppl)
Transcriptomics
ISSN: 2329-8936 TOA, an open access journal
Transcriptomics 2017
October 30-31, 2017
October 30-31, 2017 Bangkok, Thailand
3
rd
International Conference on
Transcriptomics
RNA-seq data analysis for bacterial transcriptomics
Yahaya Yabo, P Monsieurs and N Leys
Universiteit Brussel, Belgium
T
he advent of high-throughput sequencing technologies has played a significant role in the transition of biomedical research
from
in vivo
to
in silico
approach. RNA-seq has emerged as one of the technologies used in the analysis of all RNApopulations
found in a cell or group of cells and effectively dominating the existing methods used such as the microarray technology.
Several tools have been recommended for the analysis of mostly eukaryotic RNA-seq data to determine differentially expressed
genes under varying conditions or treatment groups. However, up until now no consensus has been reached on the best
analytical tool/tools to adopt in the analysis, especially for bacterial RNA-seq data analysis. This work systematically compared
different combinations of alignment algorithms and differential gene expression analytical tools with the aim of selecting the
best alignment and differential gene expression tools that will improve the process of analyzing bacterial RNA-seq data to
determine most of the differentially expressed genes under different experimental conditions with high accuracy. As a proof of
concept for benchmarking the selected tools, we used real-life, paired-end and RNA-seq reads of 150 nt length obtained from
sequenced libraries of
Cupriavidus metallidurans
(NA4 strain) using Illumina HiSeq 2000 platform. The evaluated alignment
algorithms showed comparable performance in aligning reads to the reference genome. Among the tested tools for differential
gene expression analysis, edgeR detected more number of genes while DESeq2 was found to be more stringent and tends to
prevent some low expressed genes with fold changes around the cut-off to be considered as differentially expressed genes, as
such lowering the number of false positive detections. We propose base on our benchmarking results a pipeline for the analysis
of RNA-seq data for bacterial transcriptomics.
yahaya.yabo@udusok.edu.ngTranscriptomics 2017, 5:3 (Suppl)
DOI: 10.4172/2329-8936-C1-016