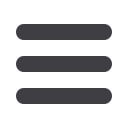
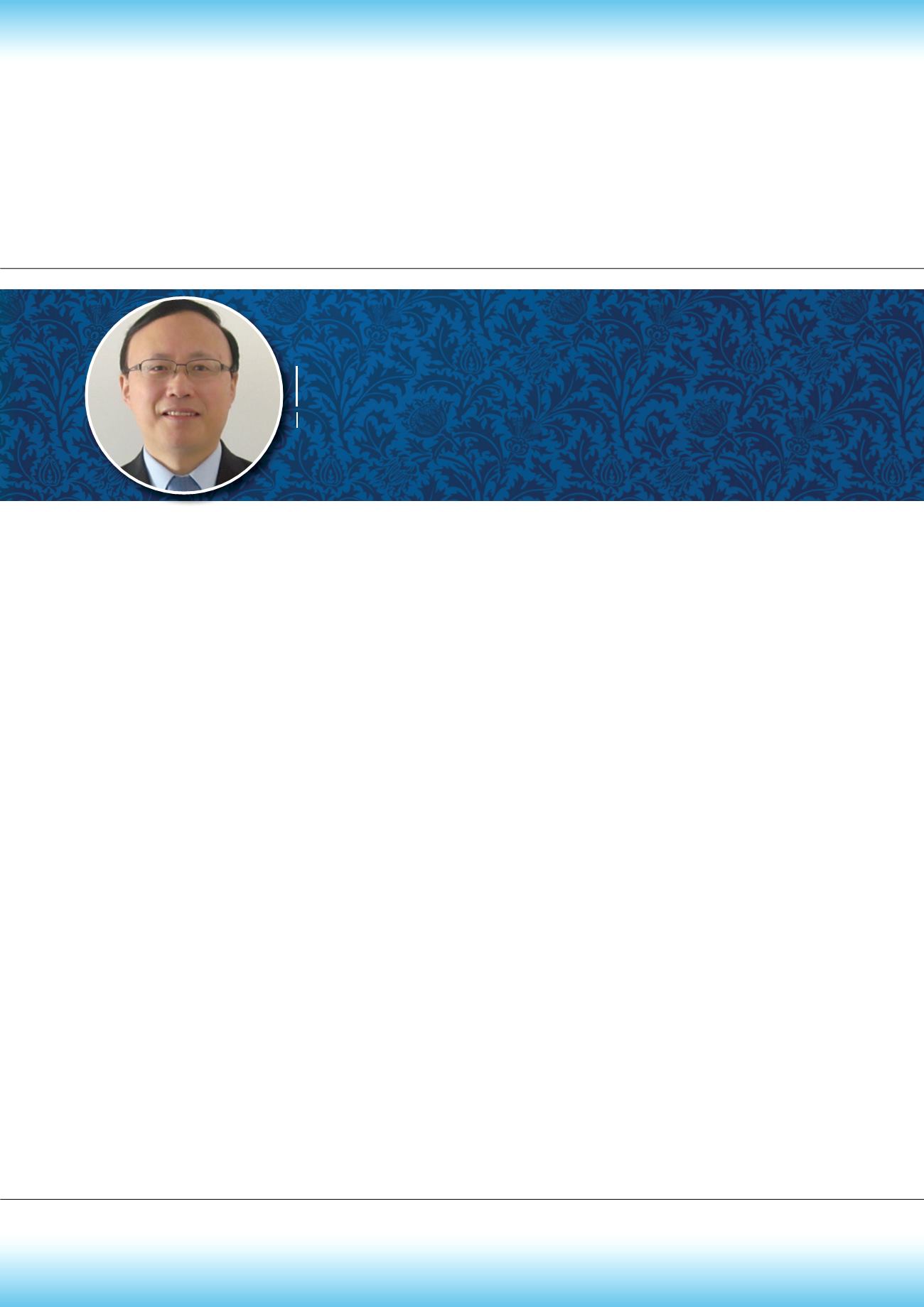
Volume 5, Issue 5(Suppl)
OMICS J Radiol
ISSN: 2167-7964 ROA, an open access journal
Page 20
Medical Imaging 2016
October 20-21, 2016
conference
series
.com
October 20-21, 2016 Chicago, USA
International Conference on
Medical Imaging & Diagnosis
Bin Zheng, OMICS J Radiol 2016, 6:5(Suppl)
http://dx.doi.org/10.4172/2167-7964.C1.009Identifying and testing new quantitative image, an analysis based clinical markers to predict breast
cancer risk and prognosis
Q
uantitative image feature analysis plays an important role in cancer screening, diagnosis, and prognosis assessment. In
our laboratory, we focus on identifying and testing new quantitative image analysis based clinical markers to help more
accurately predict cancer risk and prognosis. In this presentation, I will discuss our work and recent progress in assessing
near-term breast cancer risk and breast tumor response to neoadjuvant chemotherapy. First, we applied image feature analysis
method based on detection of bilateral mammographic density and tissue asymmetry to build a new machine learning model
to predict risk of the individual women developing early breast cancer in the near-term (i.e., within the 2 years after a negative
mammography screening). Second, we extracted kinetic image features from breast MR images to build another new model to
predict the likelihood of complete response of breast tumors to neoadjuvant chemotherapy. To increase confidence of clinians
to consider and/or accept the prediction results of our new models, we also developed and implemented the graphic user
interface (GUI) platforms for interactively applying our new image processing scheme and prediction models to process and
analyze images. Our preliminary testing results using several image datasets demonstrated that applying the new quantitative
image feature analysis basedmodels could yield significantly higher discriminatory power in predicting near-termbreast cancer
risk and tumor response to the chemotherapy. If it is successfully applied, the new image marker based prediction models has
potential to help establish a new and more effective personalized breast cancer screening and/or treatment paradigms in the
future.
Biography
Bin Zheng has received his PhD from University of Delaware in 1993. After working in Department of Radiology, University of Pittsburgh for 20 years, he joined
the Faculty in University of Oklahoma in 2013 as a Professor at School of Electrical and Computer Engineering and Oklahoma TSET cancer research scholar at
Stephenson Cancer Center. Since 1998, he has served as Principal Investigator in five R01 and one R21 grant awards from NCI, NIH, one DOD breast cancer
project, and subcontract PI of two other NIH R01 grant awards. Currently, he is AIMBE Fellow and an Editor-in-Chief of
Journal of X-ray Science and Technology.
bin.zheng-1@ou.eduBin Zheng
University of Oklahoma, USA