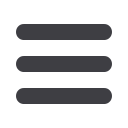

Volume 6 Issue 5(Suppl)
J Clin Exp Pathol
ISSN: 2161-0681 JCEP, an open access journal
Page 18
Digital Pathology & Pathologists 2016
December 05-06, 2016
conference
series
.com
December 05-06, 2016 Madrid, Spain
9
th
World Digital Pathology & Pathologists Congress
Christian Munzenmayer, J Clin Exp Pathol 2016, 6:5(Suppl)
http://dx.doi.org/10.4172/2161-0681.C1.027An automated microscopy system for malaria diagnosis
A
ccording to the World Health Organization (WHO), in 2015 there were an estimated 214 million cases of malaria (438000
deaths). Malaria is caused by parasites of five species, most importantly Plasmodium falciparum and P. vivax. Microscopic
examinations, the recommended Gold standard are increasing (188 million microscopic examinations in 2012 globally).
Therefore, Fraunhofer IIS is developing an automated microscopy system to support diagnosis of malaria. After automated
detection of the smear with low magnification (2.5x) a configurable number of high-power fields (63x oil immersion) are
acquired automatically. The detection algorithm starts with a custom threshold and morphologic detection step to extract
possible plasmodia candidates. These candidate objects are afterwards automatically classified to separate non-plasmodia
objects from plasmodia. For our system we evaluated two approaches. The first approach is based on a large variety of color
and color texture features, automated feature selection and a support vector machine (SVM). The second approach is based
on a deep learning scheme with convolutional neural networks (CNN). Both classifiers where trained with pre-annotated
data from 44 slides-containing mostly P. falciparum and P. vivax cases (15.499 plasmodia, 39.374 non-plasmodia). The
disjoint test set contains 37 slides with P. falciparum and P. vivax. With the SVM we reached an overall accuracy of 95.4%
(92.7% sensitivity/95.5% specificity). The CNN performed slightly better with an accuracy of 96.7% (94.0% sensitivity/96.8%
specificity). Based on these results our system helps to diagnose malaria infections in a less tedious, more secure and even more
objective process.
Biography
Christian Munzenmayer has completed his Doctorate from the University of Koblenz-Landau in 2006. Since 2000, he is with the Fraunhofer Institute for Integrated
Circuits IIS, Germany. His primary research interests center on image and texture analysis, automated microscopy and digital pathology. He is the Head of the
research group Medical Image Processing and Manager of the business field Digital Pathology and Laboratory Diagnostics. He is the author and co-author of over
60 publications in scientific journals and proceeding volumes and has been Reviewer for Medical Engineering and Physics.
christian.muenzenmayer@iis.fraunhofer.deChristian Munzenmayer
1Fraunhofer Institute for Integrated Circuits IIS, Germany